The Fourth AI Inflection
The Fourth AI Inflection
Artificial Intelligence (“AI”), and Generative AI in particular, is currently going through a massive hype cycle, but the underlying technologies have been evolving for years and are poised to drive a fourth wave of AI-driven business value in the coming years. This article covers the current state of the new AI wave, how we got here, and why the present moment is an inflection point. It will explore how companies should start thinking about driving business value from this next wave of AI as well as the practical limitations and cautions of implementing these technologies across their organization.
Authors
Sumeet Gupta, Senior Managing Director
Dr. Claudio Calvino, Senior Managing Director
Madhur Mahajan, Director
Phil Reilly, Senior Consultant
Introduction
We are in the midst of an unprecedented hype cycle. Indeed, the level of interest around ChatGPT has vastly exceeded that of the crypto mania in 2021 (Figure 1). The other recent hype cycle with the metaverse, which in the moment seemed to define the zeitgeist, is in the rear-view mirror and merely a blip compared to the wide-ranging commercial, consumer – and indeed philosophical – interest ChatGPT and generative AI have driven.
Figure 1: Hype Chart 2021-2023
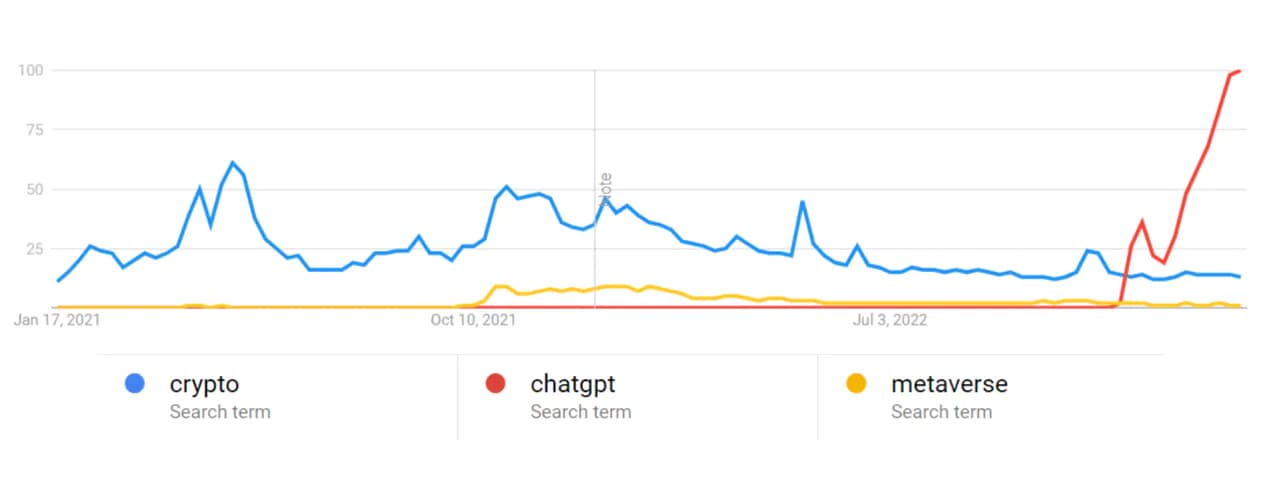
The pace is so blistering and volatile that governments have become closely involved with discussion on regulations and various groups have called for moratorium on further research till the human impact of this field can be fully understood.1 We know from history that such hype cycles are followed by an equally robust decline in mass interest, either extinguishing entirely or gradually normalizing to a period of pragmatic yet intense maturity. We know also from history the inadvisability of ignoring the economic possibilities of such events. These periods of intense maturity have created the modern digital ecosystem as we know it on the back of vigorous “tulip mania” cycles such as the dotcom hype in the 2000s.
How we got here and why the current moment is relevant
AI and the sub-discipline of machine learning, for our purposes, can be defined as the autonomous ability of systems to leverage vast tracts of data to continuously improve and automate a range a business functions from manufacturing processes to customer service tasks rivaling or exceeding human capability. This technology – first conceived and developed in the 1950s – has since gone through a trajectory that is now at the fourth inflection point.
Figure 2: Fourth AI inflection point?
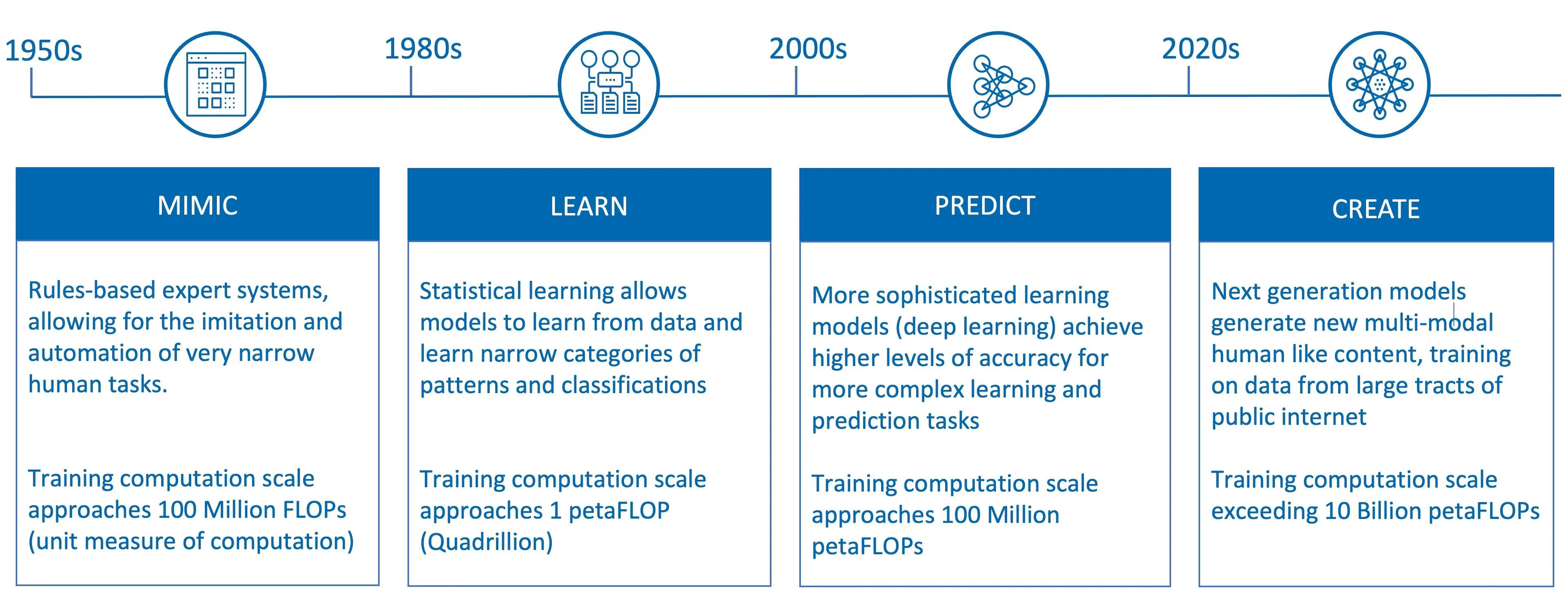
The first fifty years of this journey were dominated primarily by academic discourse and scientific research that set the foundations for the last two decades, arriving at turning point in the early 2000s with ML/AI engineering that led to a feverish increase in commercial activity and business applications. ML was rapidly integrated into many major products and services released by big tech over the last decade and has gained tremendous adoption across mainstream businesses.
We are now at a similar inflection point. It is important to understand the key factors that are rapidly converging to drive new categories of business value:
New Models: In the early 2000s, a new class of models such as long short-term memory (“LSTM”) and convolutional neural networks were invented, thereby significantly advancing functions such as natural language processing and computer vision. Similarly, the invention and production of a new class of machine learning models is driving the present inflection curve. An example of this is the transformer model — a deep-learning model first introduced by Google. This model introduces new paradigms such as the mechanism of self-attention — that allows the model to look at the whole context of an input data sequence — and the enabling of parallel processing and training AI models on unlabeled input data. This development dramatically reduces training time and allows the model to be trained on vast quantities of unstructured data. Many new-breed AI services, particularly those based on Generative AI including ChatGPT, are built on the transformer model.
Increasing Computation: Continuing increase in GPU computation available to these models has allowed them to be trained over billions of parameters (and approaching a trillion as we go to press) — orders of magnitude ahead of what was possible just ten years ago.
Expanding Data Exhaust: The last inflection was driven by the vast amount of data available from the consumer internet, and this proliferation of unstructured data from consumer and business systems — both publicly available and first-party — has only continued. The growing digitalization of physical infrastructure through sensors and IoT devices has also exponentially increased the amount of data available to be used for training large models that can be deployed across industries including those with heavy physical operations.
Democratization: The wide availability of these models and algorithms continues to expand. Major technology companies remain largely good citizens when it comes to open sourcing models, datasets and tools. Secondly, the cycle time for incorporating these models into commercially available platforms and cloud environments has decreased significantly. This improvement is allowing both startups and mainstream businesses to build new products and services with greater velocity, minimal friction and less reliance on advanced machine-learning talent than was a pre-requisite until a few years ago.
Cultural Shift: This may well be the defining feature of the new wave. The discourse around ML/AI and its practical applications has evolved from academic circles and sci-fi-enthusiast groups to corporate boardrooms now squarely into suburban living rooms. Such shifts cause a shift in adoption but also, and more importantly, grassroots experimentation of what is possible with these technologies. Indeed, we are seeing the results of this experimentation in how generative AI is creatively used in ways not anticipated in a research or corporate environment. For example, we have seen amateur teams work on training large language models on lower-computation devices, including smartphones using innovative techniques.
Regulation: The last decade was characterized by lack of regulation on ML/AI applications and an indiscriminate application of ML/AI and subsequent serious concerns on many levels. These concerns remain and are further discussed in the next section; but there is also more momentum in building regulations around ML/AI/us). For example, the U.S. Federal Trade Commission is currently considering regulations for AI in order to protect consumers and their data privacy2.
The proposed changes would mandate that companies provide transparency and explainability of their AI systems and would prohibit the use of discriminatory algorithms. While regulation is colloquially equated with restrictions, it often allows mainstream propagation of new technologies.
An AI-driven business architecture
As a framework for understanding how this new AI wave can create business value, it’s useful to think of three fundamental categories of economic activity for a business entity:
What you sell: It’s important for businesses to start examining how the products and services they sell will evolve or even get disrupted in the future. We anticipate the creation of not just new products but also new product categories. The ability to create high-quality synthetic content opens up possibilities to create new product lines and also expand the scope and power of existing ones. We expect this impact to be most immediate to digital products and services but expect this technology quickly to drive design creation of new physical products. For example, companies that own vast troves of digital assets can monetize those assets by training transformer models to create derivative digital assets from that set. This is an example of a new product that effectively allows real-time digital asset customization to a customer who may have been previously forced to select from the available assortment. Other customer-facing digital experiences, products and services will incorporate voice, video, text and image generation in creative ways to enhance product utility and improve customer experience (“CX”). Fast fashion companies are beginning to experiment with the scanning of vast product datasets to create design recommendations for their next merchandising releases.
How you sell: The customer journey from awareness and discovery to conversion and loyalty has rapidly evolved over the past decade and will continue to transform, at first incrementally and then more broadly. Chatbot driven product discovery is becoming more prominent, and companies are rapidly building add-ons for tools such as ChatGPT. Much like the way information search on the internet rapidly moved to ecommerce transactions building a channel into the shopping funnel, we expect these ML-driven utilities to evolve into a new channel leading into the selling customer service loyalty funnel. Synthetic content creation and the ability to dynamically generate personalized product copy and rich content based on customer segments will enable a new wave of customer experience personalization on steroids, increasing conversion metrics and Customer Lifetime Value (“CLV”) over time. The ability to train on and synthesize vast amounts of user-generated data and interaction history will allow businesses to generate next best interactions, summarize customer feedback, develop key actionable themes, identify risk and reduce churn.
Figure 3: A high-level framework to evaluate value-creation opportunities (illustrative, not exhaustive)
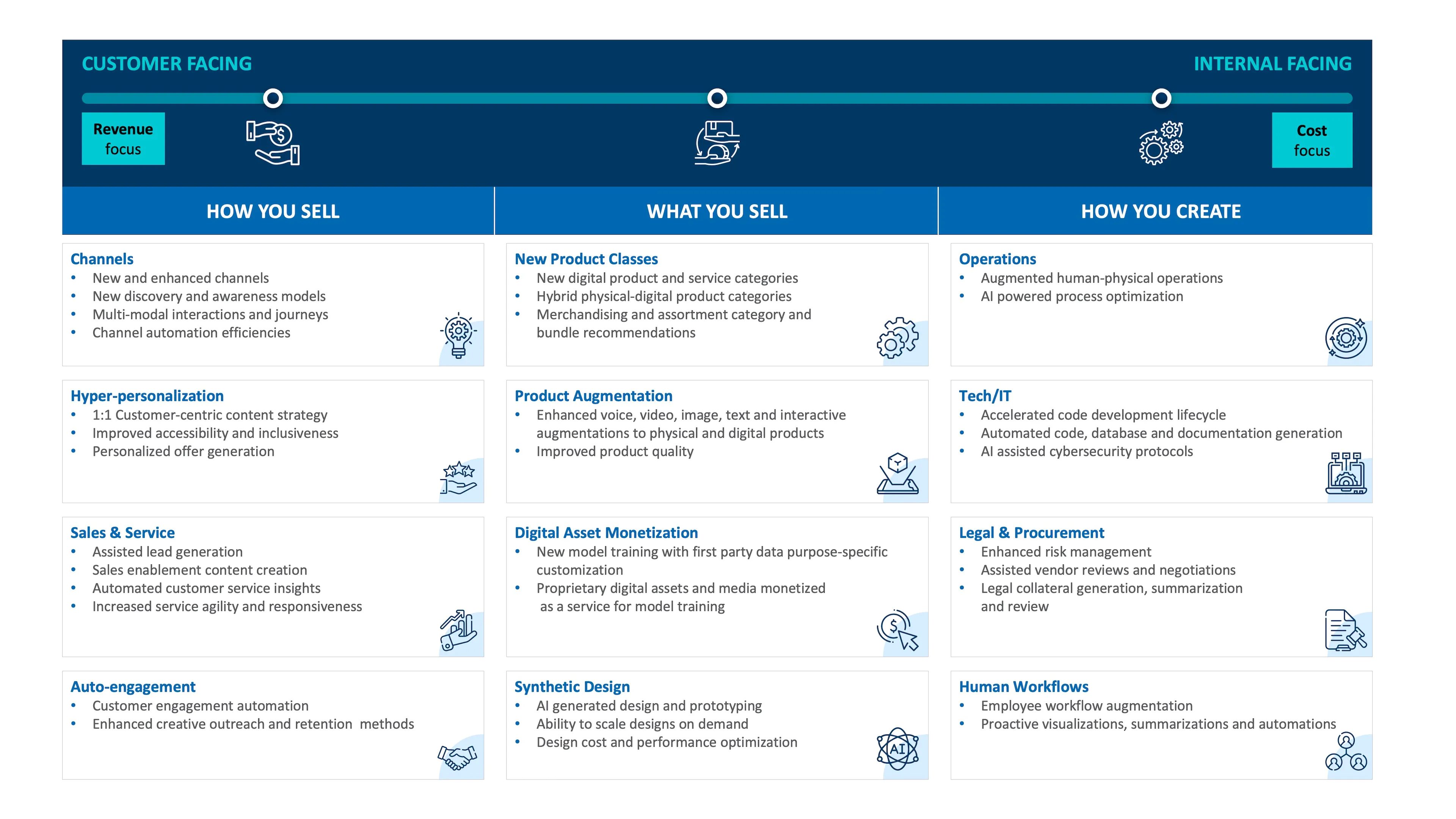
How you produce what you sell (i.e., internal operations): Intelligent automation has rapidly transformed many aspects of business operations. This will only accelerate in material ways. For example, the ability to rapidly augment code, AI-assisted database development and automated documentation generation that accelerates code to production is already gaining traction in digital and IT teams in many companies. As we go to press, numerous productivity suites are being enhanced and will change how employees work and communicate with intelligent generation of visualizations, presentation outlines, summarizations, email automation, translation, tone modifications and the like. Legal and procurement departments will soon be able to reduce the time needed to review and summarize terms, auto-detect contract clauses of interest and increase overall service levels to the business. Similarly, physical operations in industrial sectors will increasingly benefit from more sophisticated anomaly detection in plant workflows and the ability to generate human readable alerts from 3D models and images of physical facilities.
The value creation possibilities described here are expanding and materializing fast. This is evident from the level of investment and funding into substantial projects by both startups and mainstream companies as depicted in the chart below
Figure 4: Generative AI economic landscape is already expanding fast (illustrative, not exhaustive)
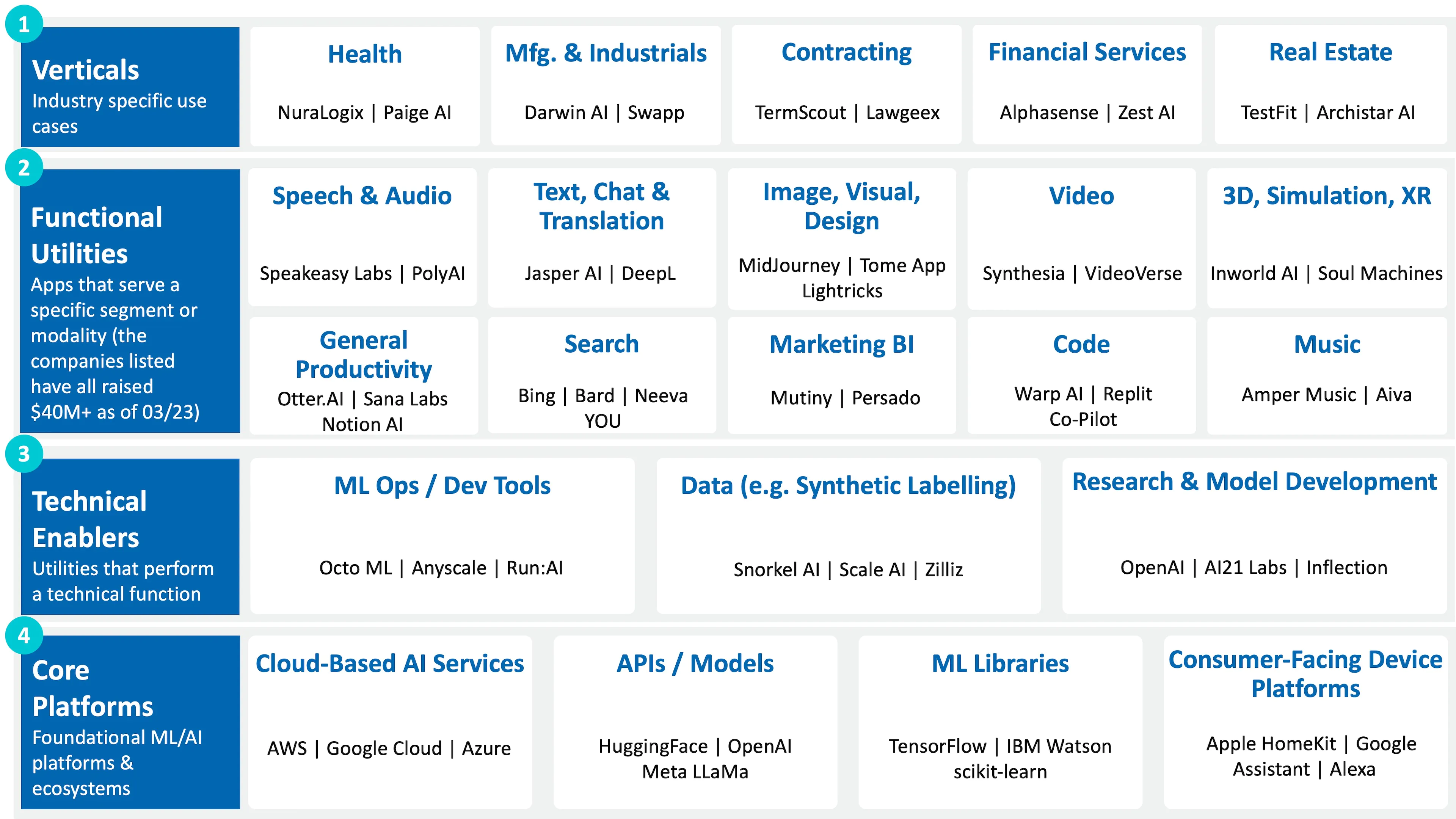
Conclusion and Cautions
AI is revolutionizing and will continue to revolutionize the way businesses operate and innovate. Like most revolutionary paradigms, it’s accompanied with many societal and ethical concerns. As businesses evaluate use-cases, it’s critical to understand and address these concerns by prioritizing the ethical and responsible development of AI:
Expensive computing: The past few decades have seen a significant advancement in computing power and resources. However, training and running AI models across your business can be computationally expensive and cost prohibitive.
Intellectual-property concerns. What is the extent to which training data can/cannot be used to generate content? Who owns derivative content? Recently, AI art generators Stable Diffusion and Midjourney were sued by an artist-collective claiming violation of copyright laws given that those tools were trained on images potentially under copyright protection.
Like humans, generative AI can be wrong. “Hallucinations” confidently generates entirely inaccurate information in response to prompts with no failsafe. AI and machine learning models are also prone to having inflated performance metrics due to issues with how the models are trained on the data, such as overfitting. The following article by FTI Consulting describes this phenomenon:
Machine Learning Model Metrics — Can I Trust Them?
The ability to moderate and filter inaccurate or inappropriate AI generated content will increasingly gain in importance.
Bias built-in to models and/or data. As evidenced in the past decade, machine learning models are heavily influenced by their training data sets. Generative AI models are no different — they are only as unbiased as the data they are trained on. Any inherent bias present in the training data will impact the output generated by the AI model. This lack of transparency poses a threat for businesses who leverage AI to inform key business decisions and can be an issue for legal or compliance purposes. The following article produced by FTI Consulting explores some of the key risks and considerations concerning AI bias, through the lens of increasing regulations within the European Union:
Mitigating Artificial Intelligence Bias Risk in Preparation for EU Regulation
Figure 5: AI Systems Bias Loop
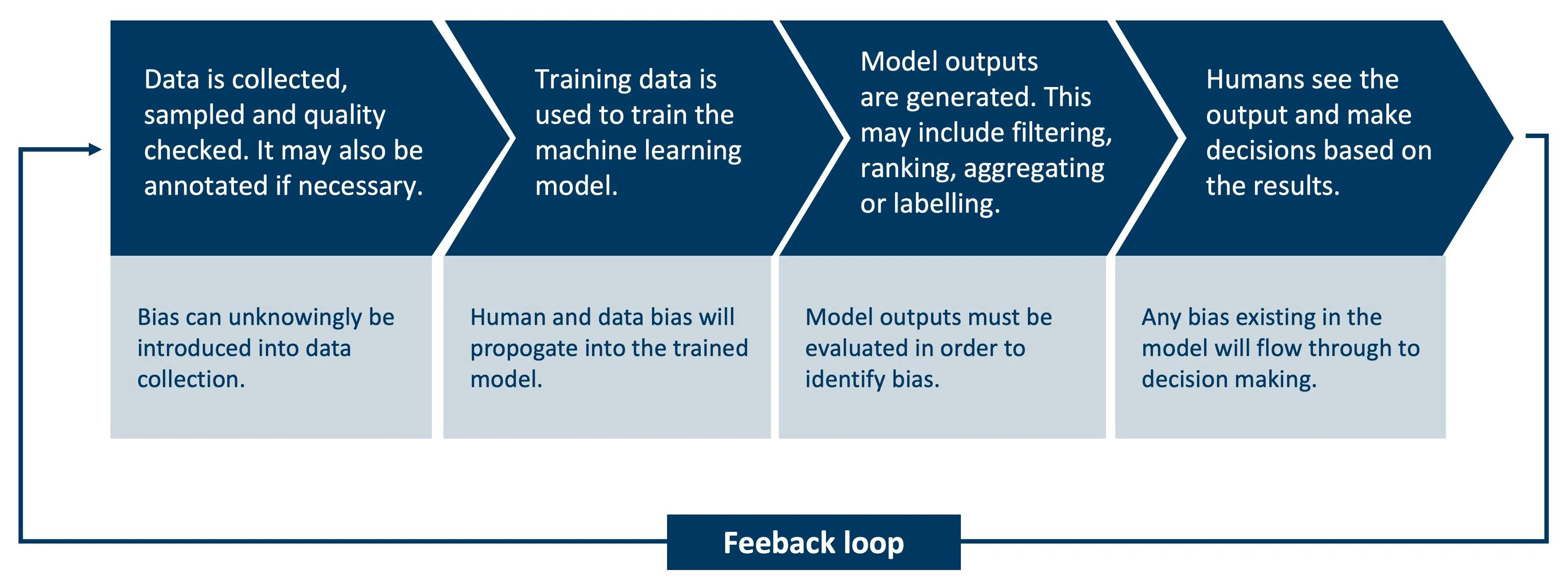
Accountability: As AI systems become more autonomous, it can become difficult to determine who is responsible when something goes wrong, raising questions of accountability and liability.
It is clear that this wave of new AI technology can be transformative for companies, and it is important for executives to begin answering these key questions in reviewing their business:
Where can the business build a competitive advantage through this new technology? And likewise, where do competitive disruptions exist across the value chain?
Based on the above issue, what is the appropriate strategic posture to adopt: pilot a close-to-cash use-case, experiment or closely monitor as a wait-and-watch strategy.
Determine how the business should establish a framework for evaluating what-to-pilot/experiments based on sound financial value.
What partners and platforms should the business invest in to allow for flexibility in these evolving AI-driven ecosystems?
How to evaluate and address the cautions outlined above.
Footnotes
“Pause Giant AI Experiments: An Open Letter,” Future of Life Institute (March 22, 2023), https://futureoflife.org/open-letter/pause-giant-ai-experiments/.
Brian Fung, “US senator introduces bill to create a federal agency to regulate AI,” CNN (May 18, 2023), US senator introduces bill to create a federal agency to regulate AI | CNN Business.